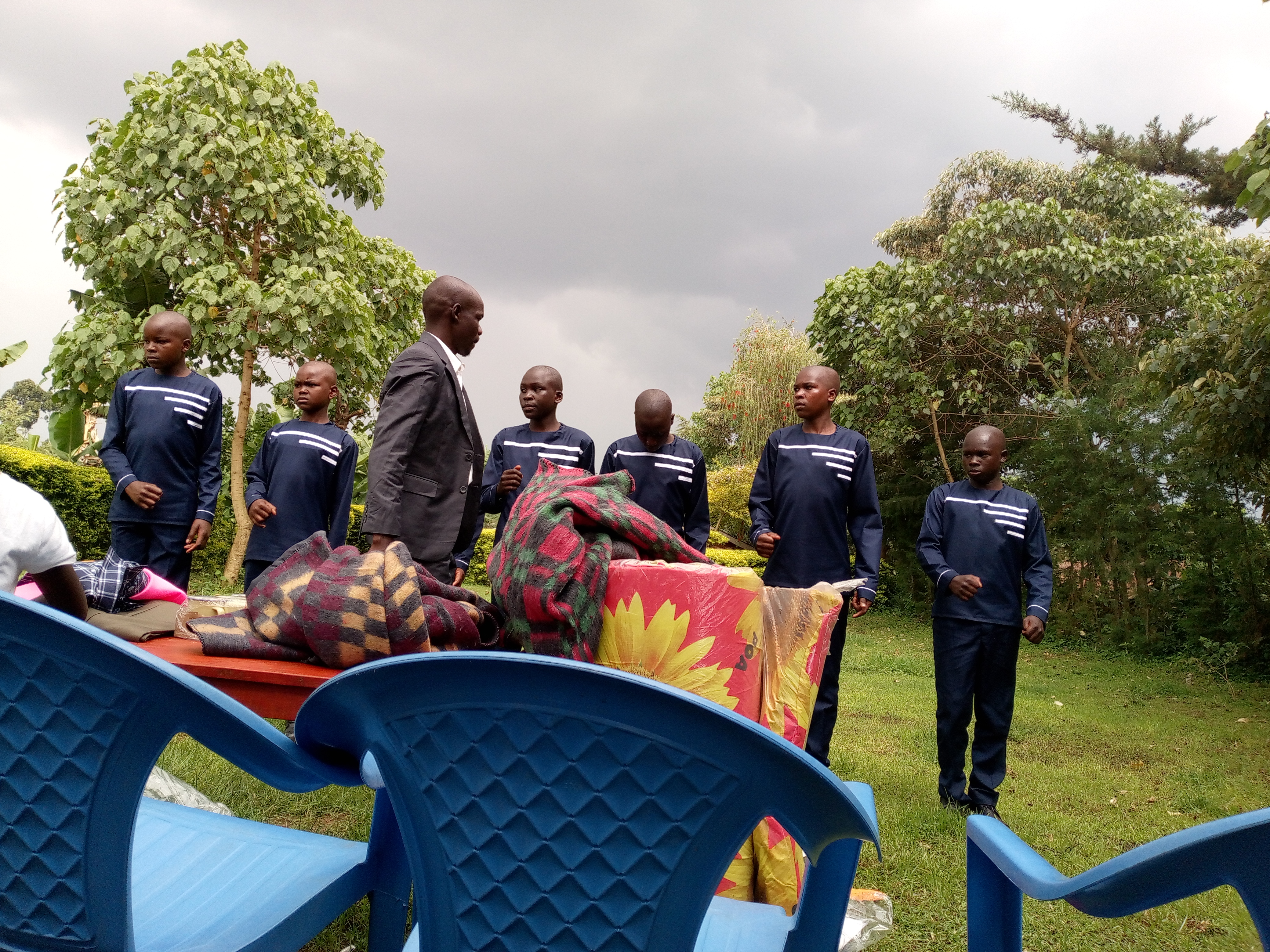
- Teacher: Ezra Kiplangat Ngetich
Moodle is an open-source Learning Management System (LMS) that provides educators with the tools and features to create and manage online courses. It allows educators to organize course materials, create quizzes and assignments, host discussion forums, and track student progress. Moodle is highly flexible and can be customized to meet the specific needs of different institutions and learning environments.
Moodle supports both synchronous and asynchronous learning environments, enabling educators to host live webinars, video conferences, and chat sessions, as well as providing a variety of tools that support self-paced learning, including videos, interactive quizzes, and discussion forums. The platform also integrates with other tools and systems, such as Google Apps and plagiarism detection software, to provide a seamless learning experience.
Moodle is widely used in educational institutions, including universities, K-12 schools, and corporate training programs. It is well-suited to online and blended learning environments and distance education programs. Additionally, Moodle's accessibility features make it a popular choice for learners with disabilities, ensuring that courses are inclusive and accessible to all learners.
The Moodle community is an active group of users, developers, and educators who contribute to the platform's development and improvement. The community provides support, resources, and documentation for users, as well as a forum for sharing ideas and best practices. Moodle releases regular updates and improvements, ensuring that the platform remains up-to-date with the latest technologies and best practices.
Links of interest:
Operating Systems is a foundational course in computer science that explores the design, implementation, and behavior of operating systems. This course delves into the core concepts and mechanisms that manage hardware resources, provide a user interface, and execute applications efficiently.
Key Topics:
Practical Applications:
Learning Outcomes:
Upon completing this course, students will be able to:
Prerequisites:
Machine Learning is a field of artificial intelligence that empowers computers to learn from data without explicit programming. This course delves into the core concepts, algorithms, and techniques that enable machines to recognize patterns, make predictions, and automate decision-making processes.
Key Topics:
Introduction to Machine Learning:
Data Preprocessing and Feature Engineering:
Supervised Learning:
Unsupervised Learning:
Model Evaluation and Selection:
Reinforcement Learning:
Practical Applications:
Prerequisites:
Learning Outcomes:
Upon completing this course, students will be able to: